As long as identity verification mechanisms exist, fraudsters will always find ways to circumvent these barriers. Among those techniques is facial spoofing (also known as face spoofing attacks), in which a fraudster tries to bypass a facial recognition system into misidentifying him by presenting a fake face (e.g. photograph, 3D-rendered models, 3D-printed mask) to the camera. Scammers can also use AI-assisted methods like deepfakes, presenting the biggest challenges for face recognition solution providers.
To protect against such threats, face spoofing detection systems such as liveness checks have evolved to mitigate emerging risks. For example, looking at the texture of the face, the density of features, and the relationship between features can all help to determine whether a face is real or not. Such technologies allow operators to gain time during the onboarding process by having a higher confidence in the true identity of their users.
As businesses in the EU and beyond continue to digitize and shift their customer identification methods towards remote KYC processes, anti-spoofing as part of facial recognition plays a crucial part in the KYC mix.
Let’s take two graphic examples to illustrate how big facial recognition is going to be:
- More and more services involved in customer-facing activities such as finance operators, iGaming websites or public entities, have started using facial recognition systems to verify their users’ identities.
- By 2023, 97% of airports plan to roll out facial recognition technology. In the U.S., 100% of the top 20 airports use facial recognition to identify international passengers, including U.S. citizens;
- And by 2025, 72% of hotel operators may use facial recognition systems to interact with their guests.
Unfortunately, the widespread use of facial recognition and the fact that sensitive businesses rely more and more on face biometrics for customer authentication led to a further increase in facial fraud attacks involving sophisticated techniques.
It is therefore even more important to understand how fraudsters operate, what methods they use, and how sophisticated and multi-layered KYC processes can minimize facial fraud.
Facial Verification methods under heavy fraud attacks
As a human paradigm, protection mechanisms are always meant to be broken.
As such, fraudsters are now looking at ways to walk-through facial identification systems by spoofing other people’s faces. For the time being, one must admit that they are talented and creative. Between static 2D or 3D attacks, deepfakes or AI-aided attacks, facial verification mechanisms are under heavy fire.
However, identification service operators also started to develop counter-measures. This cat-and-mouse game led to growing research work on machine learning techniques to solve anti-spoofing and liveness checks.
What are the most common facial recognition spoofing methods?
There can be various approaches for fraudsters looking to trick identity verification systems such as:
- Accessing buildings equipped with facial identification systems, hence potentially stealing sensitive corporate information located in those premises;
- Creating synthetic identities to register onto a service and commit other frauds (insurance scams, iGaming fraud, etc…);
- Impersonating someone’s identity (impersonation attacks);
- Avoiding screening and KYC checks or more generally, avoid being recognized by the system (obfuscation attacks)
In some cases, we observed some sort of collaboration between the “victim” and the “attacker”. Thus, while being live checked, the owner of the account who did not originate the face recognition sequence let the person through.
In order to get past facial recognition mechanisms, scammers will use face spoofing, also called presentation attacks, which can be conducted in two ways:
- Static 2D: Attackers will use any two-dimensional objects with a flat surface, like a photo or a mask, to try to impersonate a genuine user. Although the static 2D method is the most commonly used, it is also the less sophisticated, even if it is proving on a daily basis its efficiency against low-grade systems. Moreover, some found a way to mimic live movements through a sequence of pictures displayed through smartphones or tablets, and added another layer of sophistication to this attack;
- Static 3D: Attacks using 3D props such as 3D-printed masks, facial reproduction, or sculptures also found out how to tackle anti-spoofing mechanisms appealing to liveness detection of data points. Yet, there are simpler methods than others. For example, the simplest method would be to print a photograph of someone, then apply it to a deformable structure. On the other hand, the most sophisticated way would be to conduct a 3D capture of someone’s face to guarantee the highest level of details and 3D acquisition. However, rest assured that such methods request specific equipment and are very hard to setup on the fly.
In both cases, fraudsters conducting these attacks will most often use biometric publicly available data such as pictures from social networks. As people now share their pictures online more easily, fraudsters can now easily collect samples to initiate presentation attacks. In some cases, biometric data is illegally acquired on specific marketplaces.
Nevertheless, anti-spoofing mechanisms exist and can deter most of these attacks.
What mechanisms exist to tackle facial spoofing?
While spoofers deploy massive efforts to get past facial identification systems, service providers also developed an array of methods to identify presentation attacks.
The basic solution to detect these attacks is to compare the face of the user against the identity document he’s submitting. However, this mechanism can be easily spoofed and more sophisticated solutions are needed.
Thus, liveness detection provides better results to detect fraudsters. Liveness detection is the computer’s ability to determine if he is interacting with a physical person, or any other prop/video or photo presentation.
Two main domains can be drawn from liveness detection:
- Motion: motion-based methods are traditional ways to easily detect static face presentation attacks, and one of the most common methods to deter dynamic attacks.
They are divided into two sub-categories:
a. Non-intrusive: One efficient anti-spoofing technique is to detect any specific physiological sign of life from the person submitting his identity. Whether it is eye-blinking detection, facial expression changes or mouth movements, those elements provide good results, especially against static attacks;
b. Intrusive-interactive: A simple liveness detection method is to also ask the user to perform an action and to verify if it has been done in a natural way that resembles a human pattern. This method is based on the challenge-response mechanism, and is efficient against both static and dynamic attacks.
2. RPPg(PhotoPlethysmoGraphy): This methodology differs from motion-based methods, as it will not look for facial movement or expression but intensity changes in the facial skin, characteristic of pulsation in blood vessels. This method can determine blood flow only using RGB images from a distance. It can deter most attacks, although one reported weakness would be high-quality video replay attacks, because of their ability to capture periodic variation of a face’s skin light absorption.
Nonetheless, some of the liveness checks are not bulletproof, as security researchers depicted. Weaknesses have been observed during liveness detection checks, while fraudsters used high resolution monitors to present videos to low resolution cameras and fooled the identification mechanism. Therefore, it is important to carefully select a vendor complying to the highest security standards in the market.
With that in mind, more complex and robust methods reinforce the anti-spoofing toolbox.
LBP-GPM (Local Binary Pattern-Gradient Boosting Machine) and LBP-SVM (Support Vector Machine) methods can work through complex algorithms, luminance and chrominance information in order to compare two inputs. The LBP visual descriptor can also used with SVM as a classifier for quality measurement to discern between live and spoof.
Other mechanisms based on Deep Neural Network (DNN) models, involve neural networks and several neural layers to process an image, and determine if it’s real or fake.
In recent studies, researchers started exploring video-based transformers (type of DNN) for face presentation attack detection, and showed promising results. Although most approaches are binary, recent research tends to look for spoof traces by quantitatively evaluating them and reconstructing live faces. Moreover, newest trends are not focused towards presentation attacks, but are now leaning towards machine learning technologies such as Neural Architecture Search, few/zero-shot learning or domain adaptation.
Keen on learning more about Identity Fraud?
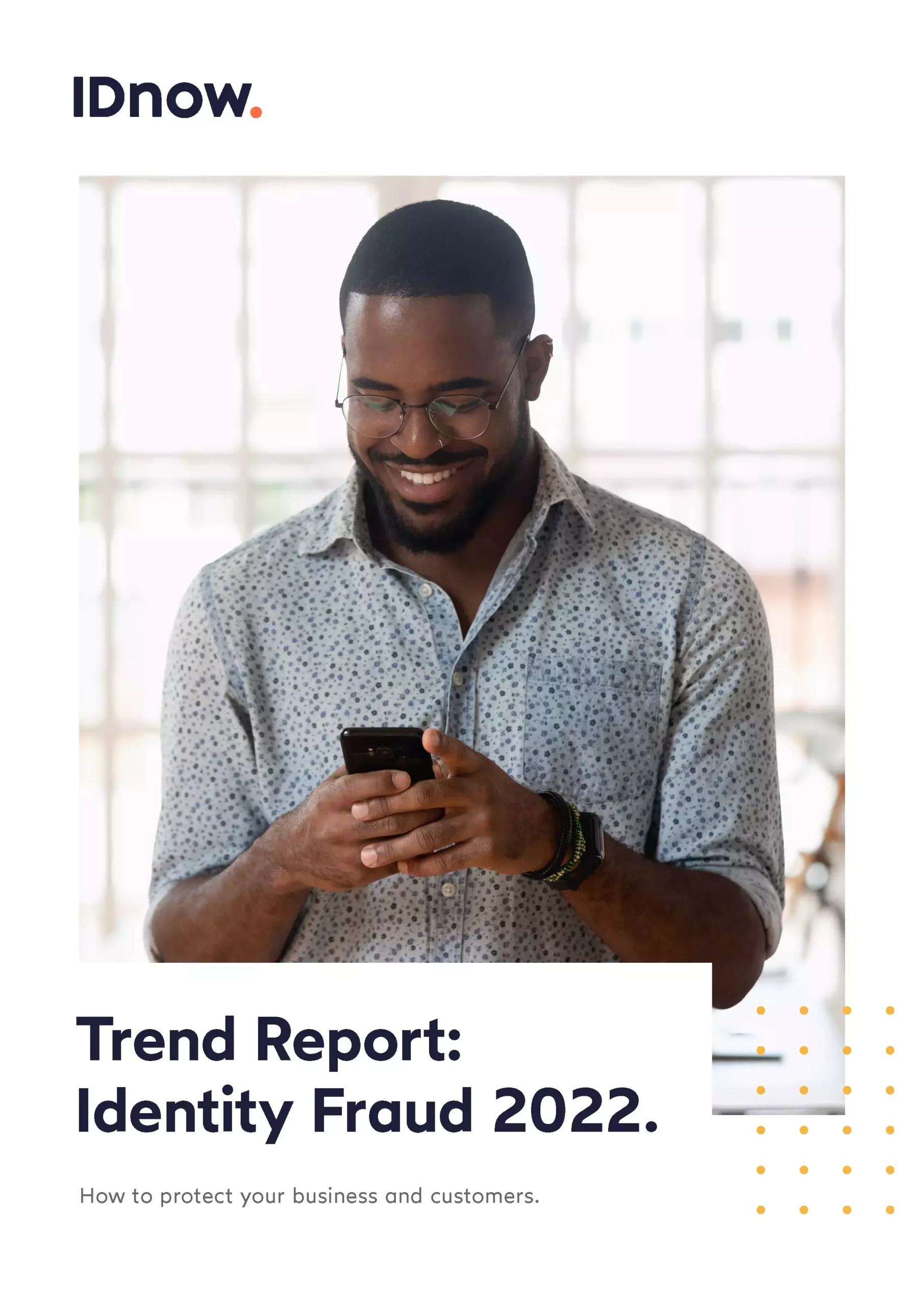
How do fraudsters try to fool the most robust recognition technologies?
If you have not heard about deepfakes, now is as good a time as any. Deepfakes have become increasingly publicized as concerns have conversely grown over their usage to create fake news. Deepfakes often refer to videos where a celebrity’s face is swapped onto someone else’s body.
They are now making their way into the identification game thanks to the progress of machine learning and availability of large-scale face databases. Identification service providers are having a hard time developing countermeasures against deepfakes, given their fast-paced evolution and sophistication level growing each day.
However, most actors from the identification services ecosystem are working and developing solutions to better detect deepfakes. Whether it is through ground-breaking methods like the one from the Facebook-Michigan State team, or through comprehensive frameworks composed of different security layers, technologies and modules, it’s always possible to prevent deepfakes.
Why biometrics solutions should be certified via FIDO
When testing against facial recognition systems, two main protocols are commonly selected: ISO 30107 or FIDO.
While both are international recognized security standards, they do not conduct security testing in the same fashion. While ISO compliance testing follows a liveness-only and a full-system mode aiming at evaluating the biometric system in different settings and against different presentation attack instruments, FIDO testing aims to test systems in laboratory settings, but also against mass population in real world conditions.
In addition, testing through ISO standards do not offer a set of metrics that would allow to obtain a “certification”, in contrast to the FIDO Alliance Biometric Component Certification. The latter presents 15 subjects to the system tested, 14 types of attack, and a total of 2100 presentations.
As a member of the FIDO Alliance, IDnow offers certified biometric checks, that complies with the highest biometric standards on the market.
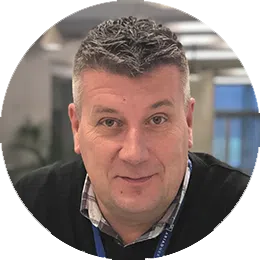
Lovro Persen
Director Document & Fraud
Connect with Lovro on LinkedIn