Sébastien Marcel, Professor and Researcher in the field of biometric security and privacy, talks to us about the rise of generative AI and the opportunities and threats it presents.
We’re hearing terms like “deepfakes” and “generative AI” everywhere at the moment, without necessarily knowing what these notions encompass. Can you explain them to us?
The notion of deepfakes can take a number of different forms:
- Face swaps: this technique allows you to take over or falsify a person’s identity by mapping two faces and inverting them. The example of Tom Cruise’s videos on TikTok speaks for itself. The impersonator uses Face swap to impersonate the actor for entertainment purposes, but unfortunately this is not always the case.
- Full image synthesis: the aim of this technique is to generate an entire image artificially. It is often used for deception, spam, phishing or to create material used to incriminate someone.
Although these two forms of deepfakes are different, they can be used as attack vectors.
Generative artificial intelligence, such as ChatGPT, can be used to generate text, images and videos. It can also be used to generate deepfakes.
Learn more about the steps we take to ensure our face verification and liveness detection capabilities are kept up to date and able to protect against deepfake attacks in our blog, ‘The liveness advantage: Transforming deepfake vulnerabilities into trust opportunities.’
What new deepfakes “trends” have you seen?
Deepfakes are becoming audiovisual. As well as constantly increasing visual quality, it is now possible to convert audio from a video. Thanks to deep learning and the creation of specific models, it’s possible to reproduce someone else’s voice. On the one hand, the video can be face-swapped, for example, and on the other, the audio can be processed separately using a similar technique, enabling us to make someone say what they didn’t say. This technique can represent a real risk in terms of fraud, especially if the fraudster manages to do it in real time.
What detection methods can be used to more easily spot these audiovisual deepfakes?
There are as yet no methods that can do this directly. For the moment, the video and audio parts can be processed separately and then combined to detect audiovisual deepfakes.
On the audio side, we’ll be looking for acoustic artifacts that might have come from a generator, such as a voice that’s a little too metallic or lacking in rhythm. These elements can sometimes fool an automatic system, but they won’t fool a human. In the future, we could also envisage exploiting multi-detection, i.e. setting up several specific detectors and making them work simultaneously to obtain the best results – this is known as ensemble learning.
On the video side, if we take the example of Face swap, we’ll also be looking for artifacts. Most of the time, videos using this technique are imperfect, and the generators leave traces, notably in the pixels of the image. Indeed, the frequency of these generated pixels is not exactly the same as in natural images. These images are too “clean” to be real, and that’s how they can also be detected.
However, with new diffusion image technologies, it is becoming increasingly difficult to detect complete image synthesis.
UK Fraud Awareness Report 2024
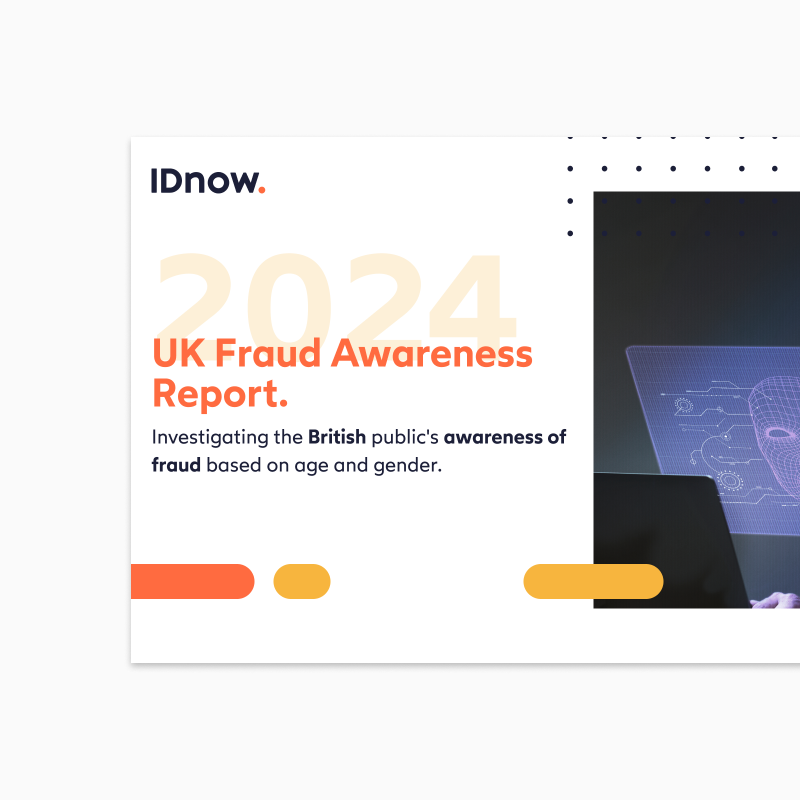
What threats do you see posed by the meteoric growth of generative AIs like ChatGPT, particularly in terms of fraud?
Generative AI is a very handy tool for fraudsters to create phishing attempts or other types of scams. On the one hand, with systems like ChatGPT we can generate well-written, realistic content that can easily fool individuals. In the long term, we can also imagine bots interacting directly with Internet users to request money or confidential data, for example.
Document fraud is another example. When entering into a remote relationship, to open an account for example, the user must submit an identity document, and, with the new tools available to us, we can now use AI generative models to create false documents with the aim of pretending to be someone else. Among the various techniques used to achieve this, morphing attacks can be used to modify a person’s identity document by affixing another photo to it, in order to bypass controls.
Conversely, what opportunities have generative AIs brought since their introduction?
These language models will become an invaluable time-saving aid for many people, particularly in the workplace. I’m thinking of people who work in the service industry. Using these models can help them optimize their time by eliminating time-consuming drafting tasks, for example.
Generative image models, such as StyleGAN, have great potential for creating synthetic data. For companies working in image or face recognition in particular, this will enable them to create realistic images to train their database, without the need to use real images, which, what’s more, are subject to a number of restrictions on the use and collection of personal data.
We mentioned earlier the risks generated by generative AI, particularly in terms of fraud. What tools or control methods do you think should be put in place?
If we take the example of misinformation in journalistic circles or on the Internet, it would almost be necessary for all images disseminated to have a label attesting to their nature, i.e. whether they have been retouched or generated entirely. This label would serve as a guarantee for the public.
Fraud attempts are more complicated, as they can come from anywhere. However, companies can protect themselves against fraud by deploying detection technologies in their customer journey. Using a service provider like IDnow to verify documents and user identities will make it easier to spot identity fraud and avoid its consequences.
OpenAI has announced the creation of a tool for the detection of AI-generated images. Do you think this will eventually make deepfakes disappear?
No, there will always be deepfakes. All we can do is try to contain, prevent and educate citizens as best we can.
How are you involved in the fight against all these types of fraud at the Idiap research institute?
At Idiap, we’ve been working on identity theft in biometrics since 2008, and we were among the first to work on the detection of presentation attacks. Then deepfakes emerged as a new technology for creating presentation attacks, for example, and we are currently working on their detection.
To fully understand how an attack works, we reproduce it ourselves, so that we can first see how it was created. We then create several attacks of the same type, in order to assess systemically and objectively whether the attack presents a vulnerability.
Finally, we create specific methods to detect attacks. The aim of these detection methods is, of course, to discourage fraudsters.
In addition to being a research institute, Idiap is also a certification laboratory. We test companies’ verification services and provide them with certificates or evaluation reports to ensure that these solutions meet a certain number of standards.
Did you enjoy this format? Discover our series of expert interviews:
- Unpacking PVID and the double-edged sword of technology, with Baptiste Forestier.
- Counting the human cost of financial crime, with Jinisha Bhatt.
- Sanctions, scams, and the elusive KYC silver bullet: FinTech Spotlight Interview with Vivek Mishra.
By
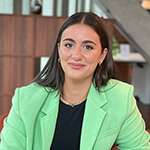
Mallaury Marie
Content Manager at IDnow
Connect with Mallaury on LinkedIn