IDnow’s collaborative research project, MAMMOTH, which explores ways of addressing bias in face verification systems, comes to an end in Autumn 2025. Here, we share a summary of the findings so far, and what it could mean for a more inclusive future of identity verification.
Face verification has emerged as an indispensable way for businesses to quickly and securely prove the identity of their customers. There are multiple reasons for its widespread adoption, including enhanced security, reduced operational costs, and elevated user experiences.
Learn more about the steps we take to ensure our face verification and liveness detection capabilities are kept up to date and able to protect against deepfake attacks in our blog, ‘The liveness advantage: Transforming deepfake vulnerabilities into trust opportunities.’
What is face verification and when is it used?
Face verification is a form of biometric technology that uses the unique features of a face to confirm an identity. In the context of remote identify verification, it involves capturing a digital image of a person’s face, often in real time, and comparing it to the identity photo extracted from a submitted identity document.
The ‘one-to-one’ matching process of face verification differs from the ‘one-to-many’ matching technique of face recognition, which involves identifying an individual from images of other individuals.
When combined with liveness detection, 3D depth analysis, and AI-powered pattern recognition, face verification can verify identity documents and the actual person behind them to dramatically reduce account takeovers, synthetic identity fraud, and impersonation attempts while creating a seamless customer experience.
From banking onboarding to airport security, face verification can transform time-consuming identity checks into seamless moments of trust. Beyond its commercial benefits, face verification offers significant social and economic advantages. It is particularly transformative in regions where many lack traditional forms of ID, providing an accessible means to verify identity and unlock services.
However, despite its widespread use, many face verification systems are still underperforming for specific demographics, such as darker skinned people.
In fact, a 2019 study by MIT Media Lab discovered that while face verification error rates for white men were just 0.8%, they jumped to 34.7% for darker skinned women.
This demographic bias isn’t just a technical flaw – it undermines the right to equal access to essential digital services, such as opening bank accounts or registering for welfare or health services.
Undertaking a MAMMOTH project.
In 2022, alongside 12 European partners, including academic institutions, associations and private companies, IDnow set out to break down these barriers of bias.
Funded by the European Research Executive Agency of the European Commission, the goal was to study existing biases and offer a toolkit for AI engineers, developers and data scientists so they may better identify and mitigate biases in datasets and algorithm outputs.
In April 2025, I attended the ‘Addressing visual bias in Biometric Identity Verification‘ webinar to share some of the findings from the project, discuss how face verification biases arise and what can be done to address them. I was excited to share a solution to this very real problem in the digital world.
What’s the problem? Skin tone bias in ID verification.
State-of-the-art face verification models trained on conventional datasets show a significant increase in error rates for individuals with darker skin tones. This is due to an underrepresentation of minority demographics in public datasets. This lack of diversity in the data makes it difficult for models to perform well on underrepresented groups, leading to higher error rates for people with darker skin tones. This reinforces the urgent need for targeted solutions that address demographic imbalance in training data.
What’s the solution? The power of representation.
Ensuring face verification models are trained on a balanced dataset of images featuring characteristics typically absent from public datasets significantly improves model performance overall. For instance, as identity card photos may undergo color transformations applied by issuing bodies (e.g., governments), skin tone plays an important role, particularly if the calibration is not optimized for darker skin tones.
This miscalibration can create inconsistencies between the captured selfie image and the individual’s appearance in the ID card photo, especially for those with darker skin tones.
This shows that training using a demographically balanced real-world dataset that mirrors the specific characteristics and variability of identity card images can ensure more accurate and fair recognition for individuals with darker skin tones.
To address this issue, IDnow proposed using a ‘style transfer’ method to generate new identity card photos that mimic the natural variation and inconsistencies found in real-world data. By augmenting the training dataset with synthetic images, it not only improves model robustness through exposure to a wider range of variations but also enables a further reduction of bias against darker skin faces.
Various experiments on public and proprietary real-world datasets reveal that fine-tuning state-of-the-art face verification models with the proposed methodology yields an 8% improvement in verification accuracy, while requiring only 25% of the original training data. In doing so, the accuracy gap between skin tone groups was reduced by more than 50%, ultimately leading to a fairer face verification system.
Incorporating learnings from the MAMMOTH project has enabled us to improve the IDnow face verification system so that we may better address ethnically diverse markets in Europe and beyond.
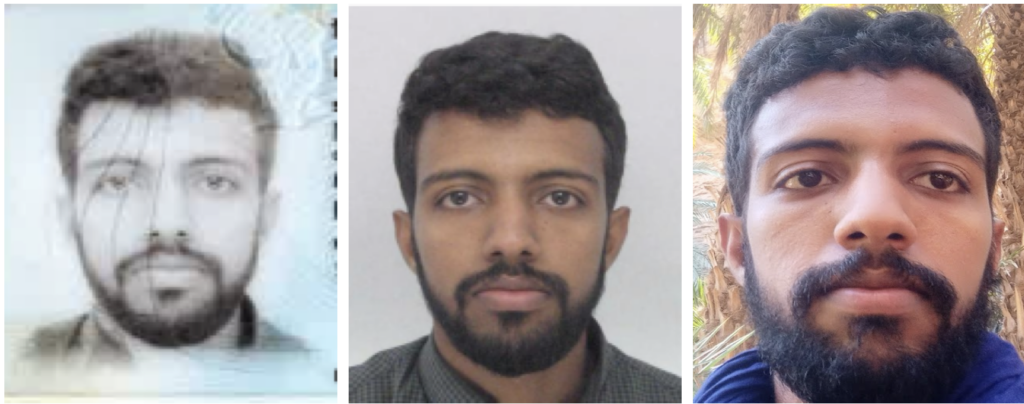
A synthetic solution to a real-world problem.
As the global adoption of biometric face verification systems continues to increase across industries, it’s crucial to ensure that these systems are accurate and fair for all individuals, regardless of skin tone, gender or age. By focusing on designing balanced, ID card-specific training datasets and leveraging synthetic data augmentation techniques, such as style transfer, we can significantly reduce bias and improve the robustness of these models.
However, without a legal framework to ensure ethical standards are adhered to, even the greatest technological breakthrough will fall short of making a long-lasting social and economic impact. We are proud of the work we are doing to reduce bias in face verification systems and are hopeful that such guidance will become standard in global regulations, such as the EU AI Act.
For a deeper dive into findings from the MAMMOTH project, watch the full webinar.
The MAMMOTH project ensured all security measures were followed to protect data and comply with internationally accepted standards and privacy regulations, including GDPR.
The MAMMOTH project was funded by the European Union under Grant Agreement ID: 101070285. Views and opinions expressed are however those of the author(s) only and do not necessarily reflect those of the European Union or European Commission. Neither the European Union nor the granting authority can be held responsible for them.
By
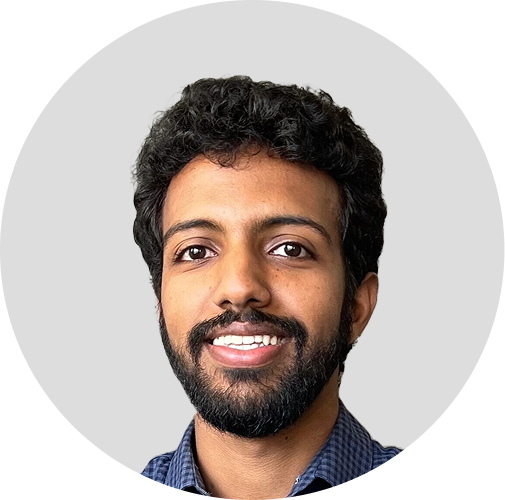
Elmokhtar Mohamed Moussa
Research Scientist, Biometrics Team
Connect with Elmokhtar on LinkedIn